Cross-modal sensory information integration in Zebrafish Olfacto-Retinal Centrifugal pathway (current)
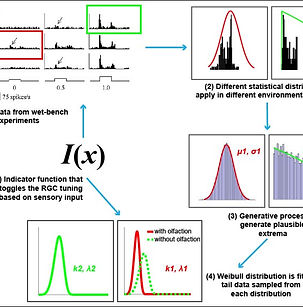
In this project, we propose a computational model of vision that describes the integration of cross-modal sensory information between the olfactory and visual systems in zebrafish based on the principles of the statistical extreme value theory. The integration of olfacto-retinal information is mediated by the centrifugal pathway that originates from the olfactory bulb and terminates in the neural retina.
Naturally, olfaction influences vision in zebrafish. A crucial observation, yielding from our own work, points to how due to olfacto-visual sensory integration, measures of visual performance or behavior in response to multi-sensory input are enhanced, when a stimulus in one modality is ambiguous or undetermined.
Image Restoration and Enhancement for Visual Recognition (current)
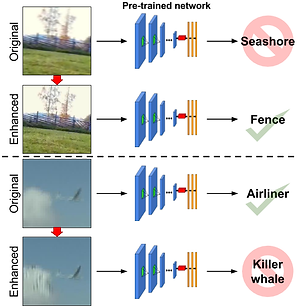
Advances in image restoration and enhancement techniques have led to discussion about how such algorithms can be applied as a pre-processing step to improve automatic visual recognition. In principle, techniques like deblurring and super-resolution should yield improvements by de-emphasizing noise and increasing signal in an input image.The goals of this project can be summed up as below:
- Analyze the impact of known problematic conditions in images collected from small UAVs.
- Evaluate the suitability of state-of-the-art image restoration and enhancement techniques from computational photography for image pre-processing before recognition.
- Collect a new benchmark data set exhibiting common imaging artifacts that inhibit recognition algorithms, consisting of public video content captured by UAVs and manned gliders, as well as controlled videos taken on the ground at Notre Dame.
​
Neuron Segmentation Using Deep Complete Bipartite Networks (2015-2017)
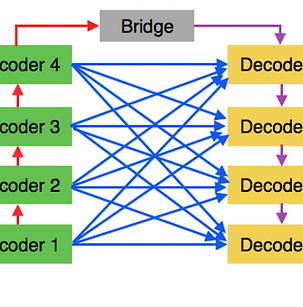
Here, we consider the problem of automatically segmenting neuronal cells in dual-color confocal microscopy images. This problem is a key task in various quantitative analysis applications in neuroscience, such as tracing cell genesis in Danio rerio (zebrafish) brains. We propose a new FCN-type deep learning model, called deep complete bipartite networks (CB-Net), and a new scheme for leveraging approximate instance-wise annotation to train our pixel-wise prediction model. Evaluated using seven real datasets, our proposed CB-Net model outperforms the state-of-the-art FCN models and produces neuron segmentation results of remarkable quality.